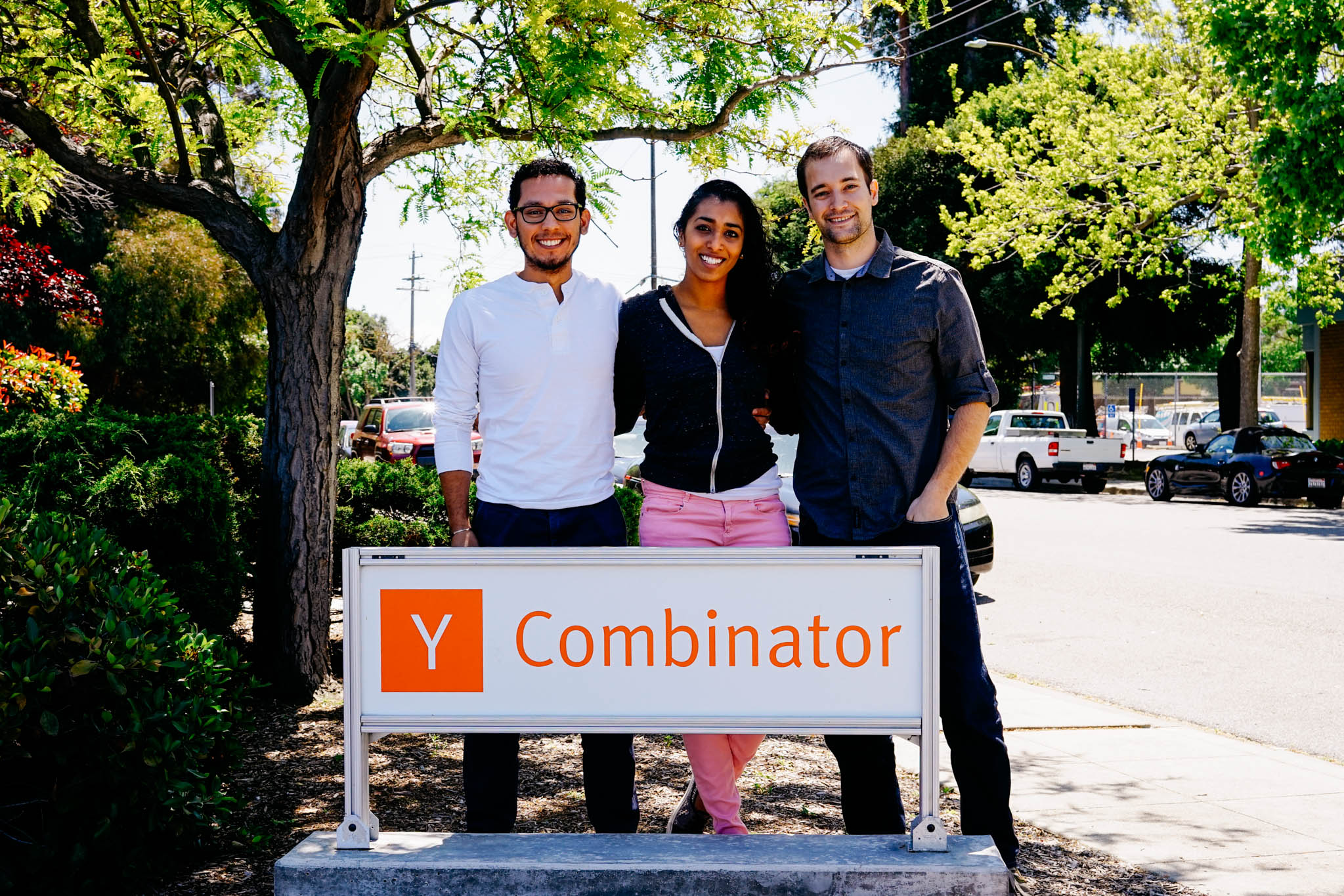
CrowdAI is building smarter image recognition. They are currently working with satellite, drone and self-driving car companies to provide them with scalable image recognition. Currently, companies spend a fortune hiring and training short-term, large-scale workforces to accomplish the same task. CrowdAI automates this process by combining machine learning, computer vision, and an efficient human workforce. We sat down with Devaki Raj, Pablo Garcia, and Nic Borensztein to talk about what they’re building.
What YC Likes About CrowdAI:
Devaki, Pablo and Nic are a dynamic team. They bring to bear the right talent, relevant experience and a high level of energy for building a great company
Tim Brady, Partner, Y Combinator
YC : How did the idea for CrowdAI come about?
Devaki: Pablo and I worked at Google for the past four years, where we saw first hand how data about the world is used for machine learning. We realized that there was so much information that still needed to be annotated. When we started talking to our colleagues about this problem, one of them introduced us to Nic.
Nic: My last startup, Ember, focused on efficiency for digital advertising. t’s an optimization problem that requires machine learning but that posed the biggest barrier for us because we didn’t have the right training data to make the algorithm run efficiently. When we got together and started talking about CrowdAI, we realized we could help companies by combining machine learning with computer vision to create high quality annotation for image data.
YC : How does CrowdAI work and how is it different from what people are doing now?
Nic: Before a company can build deep-learning models, they need to have training data. That means identifying the obstacles that are in view or understanding what objects are in the image and where they are. So, what they do is hire hundreds of workers to do this task. One company we talked to uses 600 human annotators to do bounding boxes, dense pixel labeling, and sentiment analysis. It’s a very manual and time-consuming process which is costing companies a lot of money.
Devaki: We work with data sets from self-driving car, drone, and satellite imagery companies. We make a first pass at image recognition computationally by using machine learning techniques with computer vision. About 95% of the annotation process is done through our software and the rest goes to human annotators for review. In fact we are able to offer data annotation services to companies 60% cheaper than they are able to do it themselves. All customers have to do is send us large-scale sets of images or videos that they want annotated, classified, or otherwise enhanced, and our system outputs a structured dataset that describes the contents.
YC : Is there any particular reason why you chose image annotation?
Pablo: Yeah, there’s a large need for this because machine learning algorithms for perception are not yet at the level that companies need it. Recently, there was a high profile accident using the Tesla autopilot feature because the computer’s perception wasn’t good enough. We’re seeing all these new and amazing technologies coming out that face barriers because perception is still an unsolved problem. We’re in a great position to solve this because we’re really cost effective.
Devaki: The fact that these companies are so advanced and still have to rely on human annotation is surprising. For example satellite companies send millions of dollars of hardware to space and take crystal clear images of Earth but still use humans for annotation. We help them find farms, factories, and parking lots in these images. The utility of this newly extracted data has many applications such as predicting global economic trends.
YC : Why hasn’t anyone been able to solve this until now?
Nic: It’s really three factors: neural networks, GPUs, and training data. Neural networks are finally advanced enough to be able to do these types of computation. And to do these computations effectively, you need access to scalable, GPU-based infrastructure, which is finally starting to emerge. And the last factor is the main missing piece. We’re providing them with an easy way to get training data so they don’t have to spend a lot of time and money doing it themselves.
YC : What’s the long-term vision for CrowdAI?
Devaki: We want to provide a service that annotates the world. We want to have this effective workflow where we’re pushing the amount of human annotation into smaller and smaller bits. This technology has network effects. Using the datasets clients send us, we are able to train our own perception algorithms. The more data we have the better our algorithms get. Effectively, we’ll be the go-to experts for image recognition in a variety of applications.